Exploring Machine Learning: Top 12 Advantages and Disadvantages of Machine Learning
Introduction
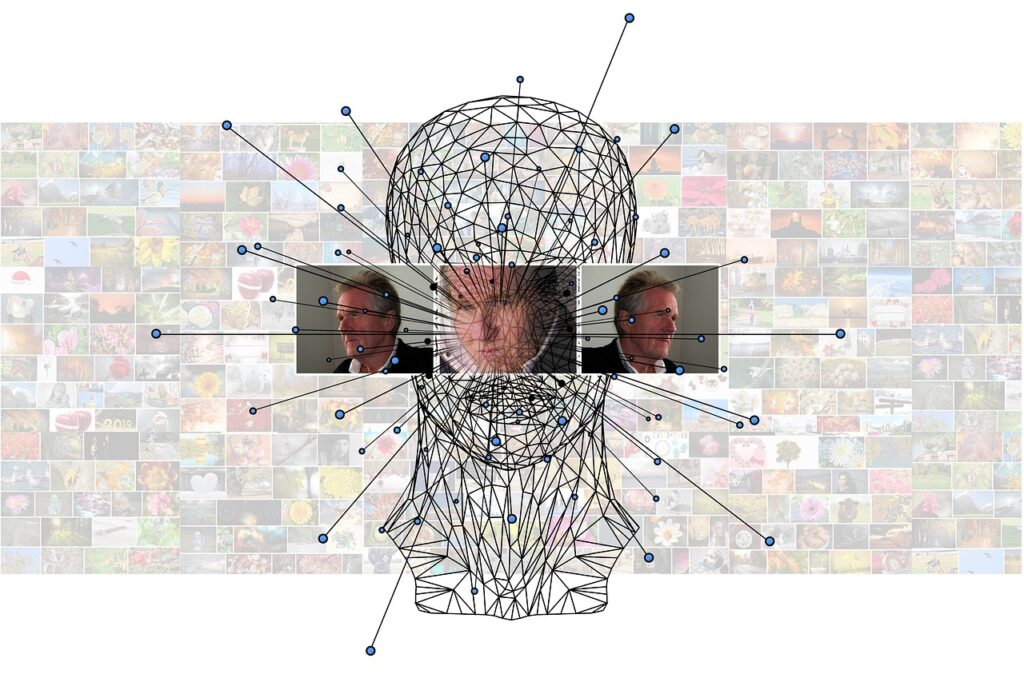
In this blog, we’ll investigate the main 12 benefits and drawbacks of Machine Learning, giving a complete outline to assist you with understanding its maximum capacity and the difficulties it presents. AI has arisen as a groundbreaking innovation, reshaping different areas via robotizing undertakings, improving direction, and offering imaginative arrangements. In any case, similar to any useful asset, it has its portion of benefits and weaknesses.
Advantages of Machine Learning
1. Automation of Tasks
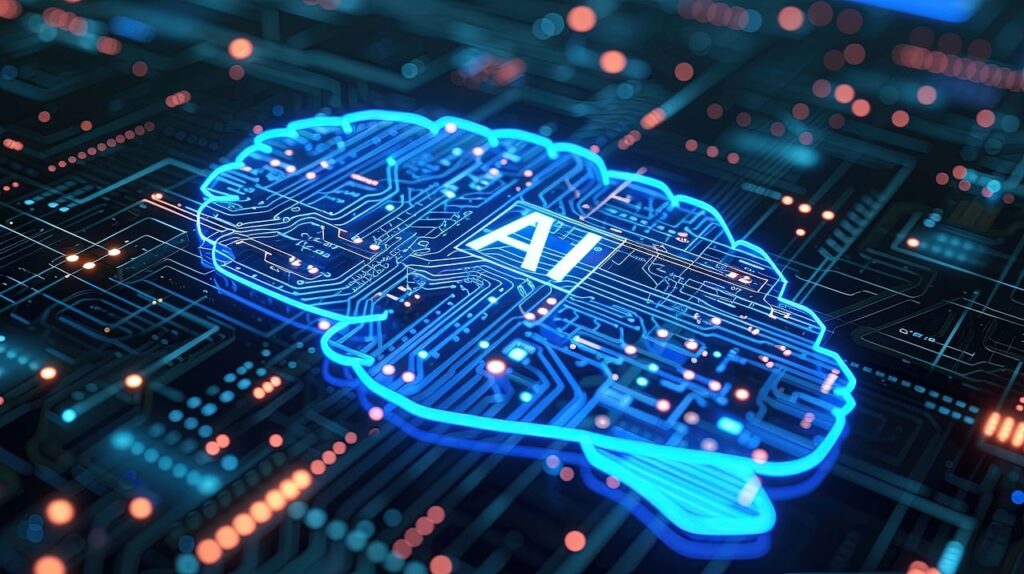
 Complex processes like extortion discovery. For example, in the assembling business, its models can screen creation lines, recognize peculiarities, and anticipate support needs, guaranteeing smooth activities and decreasing manual mediation. This increments effectiveness as well as permits human laborers to zero in on additional key and imaginative errands.
2. Further developed Navigation
One of the huge benefits is its capacity to improve dynamic cycles. Overwhelmingly of information, these calculations can recognize examples and bits of knowledge that people could miss. In the money area, for instance, such models can anticipate securities exchange drifts and upgrade venture portfolios, assisting financial backers with settling on additional educated choices. This information driven approach prompts more precise and solid results.
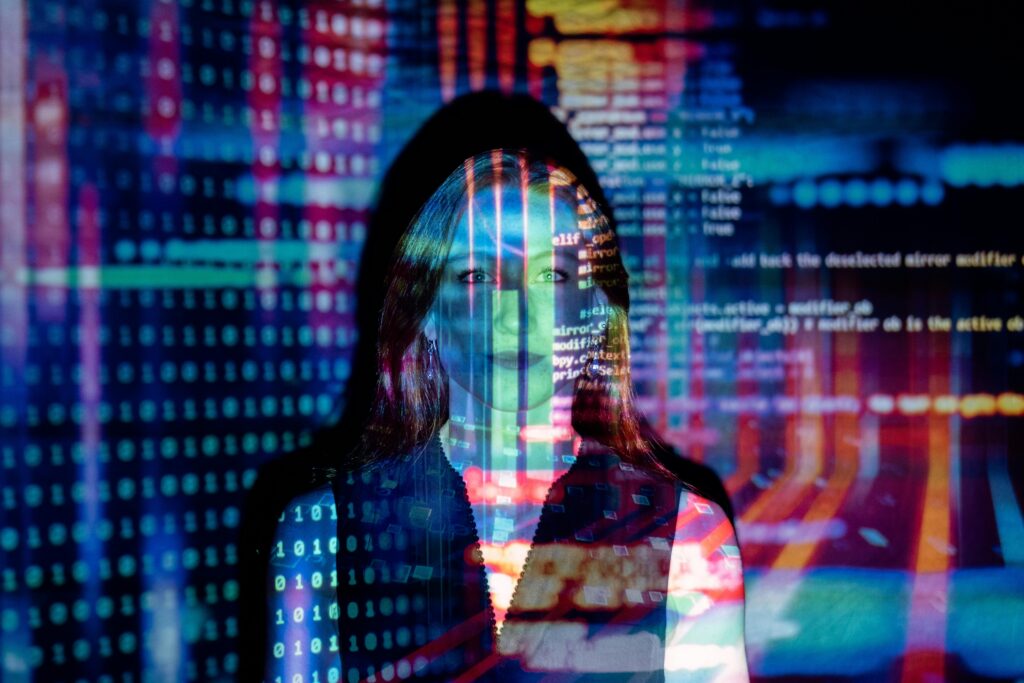
3. Improved Client Experience
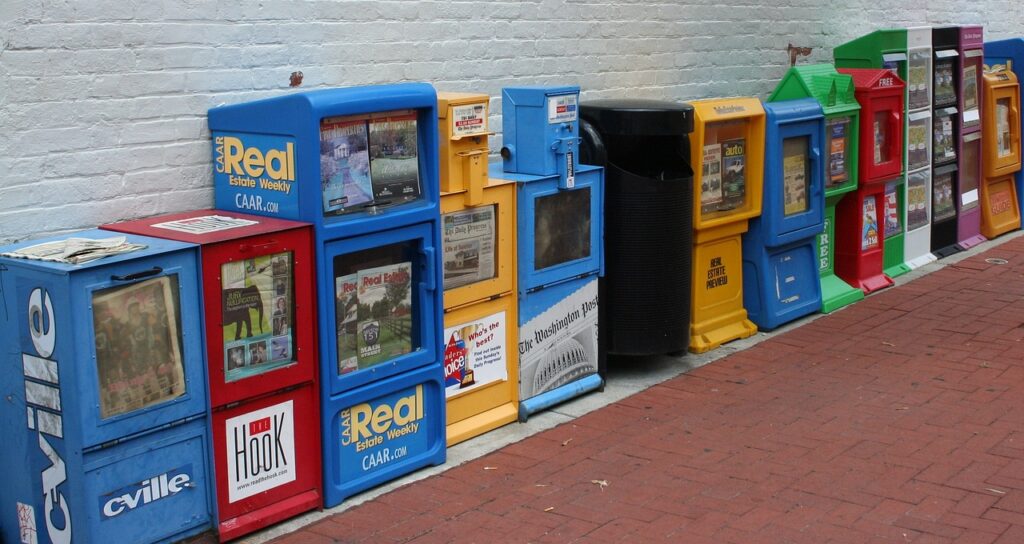
Personalization is a vital advantage of Machine Learning. Proposal frameworks, for example, those utilized by Netflix and Amazon, examine client conduct to recommend content or items custom-made to individual inclinations. This degree of customization upgrades consumer loyalty and commitment. Retailers can likewise utilize it to offer customized shopping encounters, expanding client reliability and deals.
4. Prescient Support
Prescient support is another region where Machine Learning demonstrates priceless. In ventures like assembling and transportation, these models can examine sensor information to foresee gear disappointments before they happen. This proactive methodology diminishes margin time, brings down upkeep costs, and guarantees constant tasks. For instance, carriers utilize prescient upkeep to screen the soundness of their airplane and forestall unforeseen breakdowns.
5. Misrepresentation Recognition
It is a useful asset for distinguishing deceitful exercises progressively. In the financial area, these calculations dissect exchange examples to recognize dubious way of behaving, forestalling likely extortion and improving security. These models can adjust to new extortion strategies, giving a powerful protection system to monetary establishments. Furthermore, online business stages use AI to recognize and forestall deceitful exchanges, safeguarding both the business and its clients.
6. Adaptability
Machine Learning models are intrinsically versatile, meaning they can deal with enormous datasets and adjust to new information as it opens up. This versatility is vital for organizations that arrangement with quickly developing information volumes. For instance, virtual entertainment stages use AI to examine immense measures of client created content and convey customized takes care of. As information keeps on developing, these models can scale to oblige the expanded volume, guaranteeing steady execution.
Disadvantages of Machine Learning
7. Information Protection Concerns
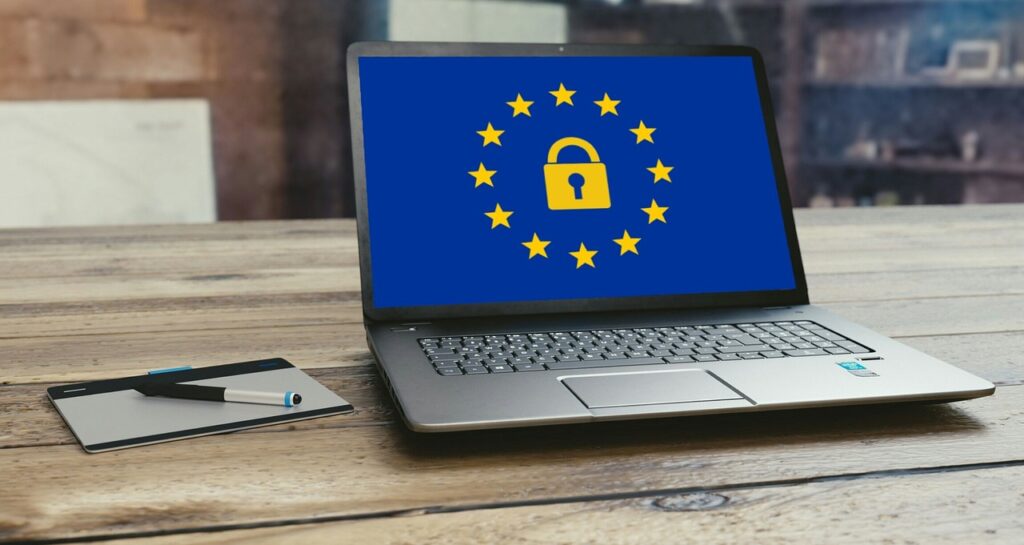
The broad information assortment required, raises huge protection concerns. Organizations should guarantee they handle information dependably and agree with guidelines to safeguard client security and stay away from breaks. Now and again, delicate data can be uncovered, prompting lawful and moral issues. It is pivotal for associations to execute hearty information assurance gauges and be straightforward about how they use client information.
8. High Execution Expenses
Creating and conveying these models can be costly. The expenses incorporate securing top notch information, putting resources into computational assets, and recruiting talented experts. For private ventures, these costs can be restrictive, making it trying to rival bigger organizations that have more assets. Also, the continuous upkeep and updates expected to keep these models successful add to the general expenses.
9. Intricacy and Skill Required
Machine Learning requires specific information and abilities, which can be a boundary for some associations. Recruiting and preparing specialists in this field can be testing and expensive. The intricacy of creating, tuning, and keeping up with AI models frequently requires a group of information researchers, designers, and space specialists. This dependence on specific ability can ruin the far and wide reception of AI in specific enterprises.
10. Inclination and Reasonableness Issues
ML models can coincidentally sustain predispositions present in the preparation information. This can prompt unreasonable results, particularly in delicate regions like employing or loaning. For example, on the off chance that an AI model is prepared on one-sided information, it might keep on going with one-sided choices, supporting existing disparities. Guaranteeing reasonableness and relieving predisposition is difficult for AI professionals. Associations should effectively resolve these issues by utilizing different and delegate datasets and executing inclination discovery and remedy procedures.
11. Reliance on Information Quality
The precision of these models vigorously relies upon the nature of the information they are prepared on. Low quality information can prompt wrong forecasts and temperamental outcomes. Information cleaning and preprocessing are fundamental stages in the ML pipeline to guarantee the honesty of the information. Notwithstanding, getting excellent information can be testing, particularly in businesses where information assortment is complicated or unstructured. Associations should put resources into hearty information the board practices to keep up with the quality and dependability of these models.
12. Moral Worries
Machine learning raises different moral worries, including issues connected with security, responsibility, and straightforwardness. For instance, its utilization in reconnaissance and policing ignited banters about the potential for abuse and infringement of common freedoms. Moreover, the absence of straightforwardness in how AI models pursue choices can make trust issues. It is significant for associations to consider the moral ramifications of their AI applications and comply with moral rules and best practices.
Conclusion
Machine Learning offers various benefits, from computerizing errands to improving independent direction and client encounters. Nonetheless, it likewise presents difficulties, including information protection concerns, high execution costs, and the requirement for particular aptitude. As enterprises keep on taking on ML, adjusting its benefits and disservices will be pivotal for expanding its true capacity while relieving gambles.
Machine Learning is digging in for the long haul, and its effect on present day businesses will just develop. By understanding its advantages and difficulties, organizations can settle on educated choices and saddle the power regarding ML to drive development and achievement. Embracing the benefits while addressing the inconveniences will empower associations to open the maximum capacity of AI and make a more effective, fair, and moral future.