Machine Learning in Manufacturing Industry – Exploring the Cutting-Edge Applications
Companies across industries are implementing machine learning to enhance their operations. It aids in predictive maintenance and supply chain optimization. With continued growth within the industry, machine learning will be a larger part of the innovation and competitive landscape.
Machine Learning for Manufacturing: Intro
Machine learning is a breakthrough technology in manufacturing. It is useful for companies since they can work faster and more productively. Using machine learning, manufacturers can gather data, identify trends, and make informed decisions. It has lots of potential to transform the industry and is already being used in numerous domains, such as inventory management and quality control.
Key Takeaways
- 61 percent of the companies in manufacturing are using machine learning for efficiency and productivity.
- Machine learning can improve the quality of products and lower costs for the manufacturing sector.
- Machine learning has been used in various industrial applications like predictive maintenance, supply chain optimization, quality control, etcetera.
- Some algorithms are capable of heuristics based on the data they process.
- ML implements for the Mfg marketplace, stirring the era of unparalleled innovativeness and competitiveness.
- The scope of machine learning can end to end in manufacturing from the design phase to the delivery phase.
Machine Learning and How to Integrate it into Modern Manufacturing
Companies in the MGF industry are using ML to analyze data from sensors and machines. This helps them predict when equipment might fail, reducing downtime. This has greatly improved production efficiency and product quality. ML is also used in supply chain management and inventory management.
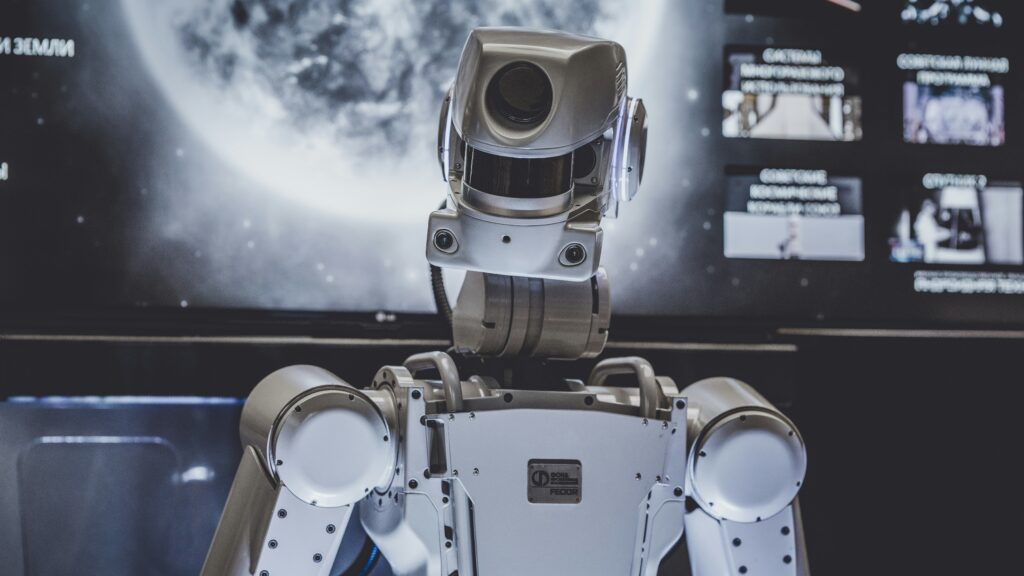
Machine learning has a huge future in our industry. This enables smart manufacturing, which can enhance efficiency and productivity. In manufacturing terms, it employs algorithms to enable machines to perform tasks that require human intelligence. This technology could make a game-changing difference: better production, higher-quality products, and lower costs.
The emergence of smart manufacturing is driven by emerging technologies such as the Internet of Things (IoT), big data, and cloud computing. These tools can take a lot of data and aggregate it. Manufacturing is improved as this data gets machine learning models trained on it. Here are some of the important tech for machine learning in manufacturing:
- IoT sensors and devices
- Big data analytics
- Cloud computing platforms
- Machine learning artificial intelligence algorithms
As manufacturing continues to change, machine learning will become increasingly important. Using smart manufacturing and key technologies, manufacturers can be better, cheaper, and more high-quality. Machine learning may revolutionize the world of manufacturing and foster economic growth.
There are many benefits in understanding machine learning revolving within contemporary manufacturing systems. It allows makers to begin to incorporate smart manufacturing into their workflows. With the right technology and expertise, machine learning can work for makers. In this way, they can remain competitive in a challenging market.
ML Applications in the Manufacturing Industry — The Current Stage
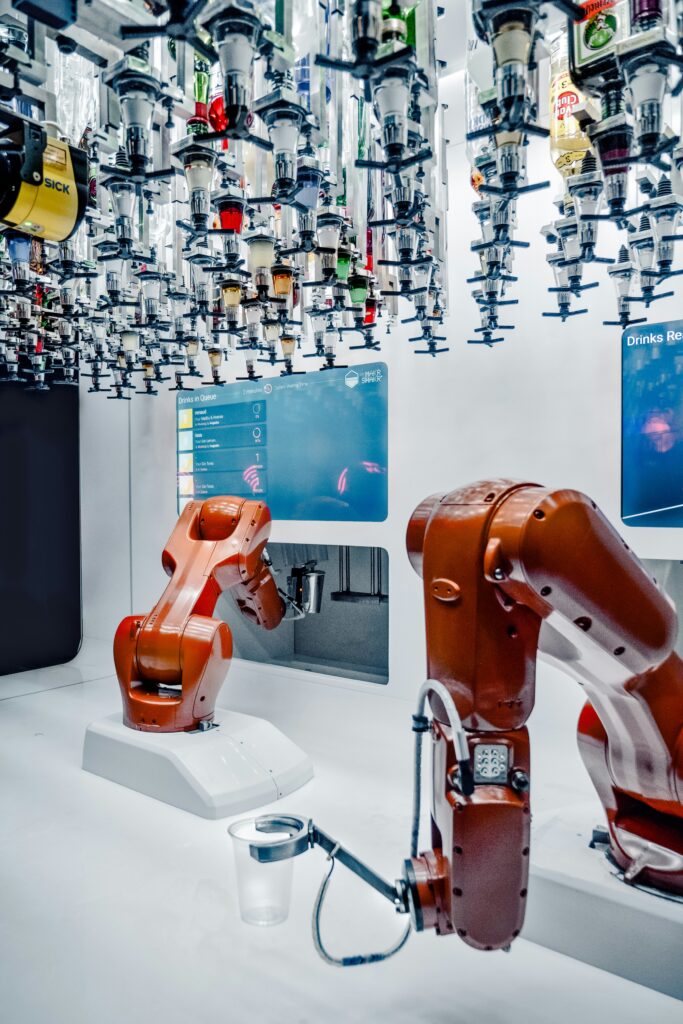
Machine learning in the MGF space is changing rapidly. ML is being deployed in several sectors to optimize efficiency and productivity. Machine learning is used in a variety of scenarios, including predicting machine downtime, enhancing product quality, and more.
MGF industry companies are applying ML in analyzing data from machines and sensors. This allows them to predict when equipment is likely to fail, minimizing downtime. This has immensely enhanced the efficiency of the production process and the quality of products. Supply chain and inventory management also use ML.
- Predictive maintenance
- Quality control optimization
- Production line automation
- Supply chain management
- Inventory management
The state of ML in the most recent year showed increased ML adoption and investment across MGF companies. With the maturation of the industry, there will be many more innovative applications of ML in MGF.
Industry Sector | ML Applications |
Automotive | Predictive maintenance, quality control optimization |
Aerospace | Production line automation; supply chain management |
Consumer Goods | Inventory management and demand forecasting |
Machine Learning Transforming Production Efficiency
Machine Learning Revolutionizing Manufacturing and Everyone Else It enhances the efficiency of production for many. This can be seen in predictive maintenance, quality control, and automating production lines. Factories use machine learning to perform better, halt production frequently, and produce more goods.
This is where predictive maintenance comes into play. It uses machine learning to predict when machines are destined to fail. This allows the factories to schedule maintenance, which minimizes lost time. Quality control, too, is aided by real-time checks. This nabs issues early, improving products and conserving resources.
Systems for Predictive Maintenance
Machine learning is used in predictive maintenance systems to analyze sensor data. They predict when machines might break down. This allows factories to schedule maintenance, minimizing lost time and improving production efficiency.
Quality Control Optimization
Enhancing quality control with machine learning. It monitors production in real-time, identifying problems. This allows factories to quickly remedy problems, improving products quickly and preventing wasted resources. Focusing on quality control can also help factories reduce rework and scrap, which saves time and money.
Production Line Automation
The automation of production lines is another major victory. This makes these processes more seamless, requiring less human intervention through machine learning. That includes things like assembling components, inspecting them, and packing them up. By automating these steps, factories can operate more efficiently, save on labor costs, and produce more stuff.
Machine Learning Based Supply Chain Optimization
Supply Chain Optimization Today and The Role of ML It analyzes historical data and current trends to predict demand. This allows businesses to manage their logistics and stock more effectively, saving money and working more efficiently.
It is important to have good demand forecasting. It allows companies to prepare for demand shifts. This allows them to serve customers well and not be overstocked or understocked.
There are a lot of advantages to applying machine learning in supply chain optimization. These include:
- More accurate forecasting
- Better logistics management
- Optimal inventory levels
They do this by making supply chains more fluid, thus enhancing customer satisfaction. They also gain a head start in the market. As machine learning is more prolific in supply chain optimization, we can expect improved logistics and demand forecasting. As a result, it will improve supply chain management.
Quality Assurance and Defect Identification
In making things, machine learning is instrumental in improving quality assurance and defect detection. It employs visual inspection systems to identify defects in products. This improves products in general. Product images are captured through cameras and sensors, then machine learning detects defects.
This is the most critical aspect in making things which is good quality assurance. It prevents bad products from reaching customers. A big part of this is defect detection, and that is improved by machine learning. It allows makers to address issues on the spot.
Global advantages of Machine Learning in quality assurance and defect detection are as follows:
- Products are better
- Less waste and scrap
- The act of optimizing speed and efficiency
- Happy customers
Visual Inspection Systems
With cameras and sensors, visual inspection systems capture images of things. Machine learning is used to examine these pictures for issues.
Real-time Quality Monitoring
First, there is real-time quality monitoring, where sensors and cameras track products throughout the manufacturing process. This allows makers to identify issues immediately and address them quickly.
Defect Classification Algorithms
Defect classification algorithms categorize defects into multiple categories such as scratches, cracks, etc. This enables makers to identify the source of issues and prevent them from recurring.
Defect Type | Description | Causes |
Scratches | Superficial scratches or blemishes | Improper handling, transit, or production mistakes |
Cracks | Small cracks or fractures | Material defects, stress, or fatigue |
Other | Other defects can give rise to holes or abnormalities | Depending on the case, material defects, manufacturing errors or design errors |
Predictive Maintenance and Equipment Health Monitoring
In today’s manufacturing environment, predictive maintenance is critical. It allows companies to monitor the health of their equipment in real time. They can even predict when something is likely to break down. That way, they can schedule when to patch things up, without surprise crashes.
Here we’d talk about some major advantages of predictive maintenance:
- More work is done because there is less downtime
- Equipment operates better and lasts longer
- It saves money by reducing the need for repairs
This requires monitoring systems. They provide real-time performance data on how well equipment is performing. This information allows you to proactively identify issues to keep things running smoothly. Smart tech like machine learning and IoT sensors is used in effective predictive maintenance.
Predictive maintenance and monitoring of the health of machinery can keep manufacturers ahead of the curve. It also boosts their efficiency and cuts costs. Predictive maintenance is going to become increasingly important as the world of manufacturing continues to evolve. It will make new, more interesting ways of working possible.
Energy Management and Sustainability Solutions
Therefore, the manufacturing industry is undergoing a rapid transition. Focusing on energy management and sustainability is the key. Machine learning enables organizations to consume less energy, thus reducing their impact on nature and using assets more efficiently. This allows them to make informed decisions about energy usage, helping to minimize waste and increase efficiency based on real-time energy consumption information.
Some key energy management and sustainability practices include:
- Instead of predictive maintenance to minimize energy waste and equipment downtime
- Rescheduling production to reduce power usage at peak times
- Investing in renewable energy sources, such as solar or wind power, to reduce reliance on fossil fuels
Reduce Environmental Impact Manufacturers just need to focus on energy management and sustainability to reduce their environmental impact and save money. Better energy management leads to reduced energy expenses and improved corporate image. It enables them to catch up in a quickly evolving industry – and cranks up a lighter footprint of production.
Machine learning plays a crucial role in the manufacturing sector in energy management and sustainability. Through power consumption optimization and resource utilization enhancement, businesses can now expect a more efficient future with a lesser impact on the environment. They will also practice energy management best practices in their operations.
Use Cases of My Data Science Notes in Supply Chain
Good inventory management and demand forecasting is essential for an effective supply chain. Machine learning analyzes past sales, seasonal trends, and NA data for providers. In this way, they can forecast demand and manage their inventory much better. It helps prevent stockouts, reduces waste, and keeps customers happy.
There are many benefits of using machine learning for these tasks. For example:
- It increases demand forecast accuracy
- It allows you to better manage inventory levels
- It minimizes stockouts or excess stock levels
- It improves the supply chain efficiency
Integrating inventory management and demand forecasting into the supply chain enables businesses to become leaner and more dynamic. They can respond quickly in a changing world of demand, supply, or market factors. These result in better competitiveness and profits.
The data-driven solution to the complex world of inventory In a world with increasingly complex supply chains, efficient inventory management, and demand forecasting can be the difference between success and failure. Many businesses will be able to stay ahead by utilizing machine learning and other advanced tech. That they can win long-term in a competitive market.
Separation of humans and machines instead of human-machine collaboration
The manufacturing world takes worker safety very seriously. With our data and training cut off in October 2023. Human-machine collaboration is critically important to ensuring everyone remains safe. And with more sophisticated machines working, safety systems are crucial to prevent accidents.
Technology makes work safer and better for humans and machines. Safety monitoring systems monitor for perils and alert workers. This allows everybody to work together in safety.
The safety systems watch things via sensors, cameras, and alarms. And, for example, they can detect whether someone is too close to a machine. This protects everyone and teaches essential safety skills.
Safety Monitoring Systems
- Production lines and surrounding environments can be monitored in real-time
- Identifying potential hazards and warning workers to take proper precautions
- Developing safety protocols and procedures to avoid injuries and accidents
Collaborating with machines is also critical for safety. Regular training provides the workers with proper knowledge of operating machinery. Which includes which operating system to use and what to do in the event of an emergency.
Emphasis for Human-Machine Teaming as Safety Needs Evolve For a safe work environment, safety monitoring is very critical.
Reduce Cost And Optimize RoI
Machine learning reduces costs in manufacturing by discovering and eliminating inefficiencies. It sifts through enormous amounts of data to predict when things need repair, helping to reduce manufacturing costs.
Here are five machine learning-enhanced approaches that deliver savings and improve returns:
- Predictive maintenance is used to minimize downtime, and repair cycles, and extend equipment life
- Media caption It follows concerns of quality that wiped £395m off the manufacturer’s share price in October
- Streamlined logistics and transportation cost reduction through supply chain optimization
Machine learning allows makers to save money and generate more revenue. Companies that use it have reduced their manufacturing costs by 10-15%, according to reports sweeping over several major companies.
Machine learning is changing the manufacturing game. It will bring large opportunities for cost savings, increased revenue and improved efficiency. And as it improves, we will see more interesting applications of machine learning in creation.
Advantages of Machine Learning in Manufacturing | Description |
Cost Reduction | Identification of areas of inefficiency and optimization of production processes |
ROI Optimization | Predictive maintenance and minimized downtime |
Enhanced Efficiency | Optimization of logistics and decrease in transportation expenses |
Challenges of Implementation and Their Solutions
The use of machine learning for manufacturing often suffers from implementation challenges for companies. Such obstacles create challenges in integrating machine learning models into existing systems. One of the huger concerns is technical integration — ensuring the new technology integrates with existing technology seamlessly.
Workforce adaptation is another big challenge. They need to pick up new skills as machine learning takes some tasks off their plate. That can be hard, especially for starting companies or major transitions.
- Create a full implementation plan for technical integration, and people integration.
- Gather data from other contexts or historical events to learn lessons that are relevant today.
- To do this, it is best to establish direct lines of communication among all involved parties.
Challenge | Solution |
Technical Integration | Establish a foundational integration plan |
Workforce Adaptation | Train and support workers |
Data Security | Establish robust security protocols |
Organizations that solve these problems and find the right approaches can make machine learning effective. This ensures efficiency, productivity, and a leading edge in the market.
Case Studies: Successful ML to Manufacturing Implementations
There are many case studies about the impact of machine learning on the manufacturing industry. They have observed improved efficiency, reduced costs, and enhanced product quality.
Some examples include:
- Predictive maintenance systems that identify equipment faults early, reduce downtime, and increase productivity.
- Machine learning enhances quality control by spotting defects and irregularities immediately.
- Machine learning makes supply chains smarter by predicting demand and managing stock.
So, a leading research firm in the manufacturing industry found something pretty interesting. Machine learning-powered companies had 10% higher productivity and 5% lower costs. And this depicts the strength of machine learning in manufacturing.
Manufacturers can learn a lot from examining these case studies. They can discover how to apply machine learning in their own work. This translates to better results and greater success in manufacturing.
Company | Implementation | Results |
Company A | Predictive maintenance | 10% increase in Productivity |
Company B | Quality Control Optimization | Decrease in defects by 5% |
Company C | Supply Chain Optimization | 3% cost savings |
The Future Trends and New Technologies
The manufacturing sector is poised for a major shift. This transformation is being influenced by future trends and new technologies. Now, advanced analytics will play a critical role in setting the industry’s trajectory forward.
Here are a few key areas to pay attention to:
- Integration of Advanced Analytics: This will allow manufacturers to gain better insights and make sounder business decisions.
- Edge Computing Applications: Processing data close to the source allows the manufacturers to take faster decisions in real-time.
- AI-ML Hybrid SystemsIMore specialized & automated systems which will result from dissecting AI and ML
As these emerging technologies mature, we’ll see substantial increases in efficiency, productivity, and innovation. Manufacturers standing at the forefront of the fast-evolving pace of modernization, by embracing trends of the future and adapting to the advanced analytics model can switch their manufacturing navigation to the infinity phase.
Technologies | Description | Advantages |
Advanced Analytics | Use of automated data analytics and machine learning | Enhanced decision-making, enhanced efficiency |
Edge Computing | Analyzing data near the data source | Decreased latency, enhanced real-time decision-making |
ML Hybrid Systems | Integration of artificial intelligence and machine learning | Improved autonomy, increased productivity |
AI-ML for Manufacturing: Best Practices for Integration
Best practices need to be followed while integrating ML into the manufacturing industry. This involves preparing your data, selecting appropriate models , and employing them correctly.
How Manufacturers Should Adopt ML: A Structured Approach This includes:
- Defining the clear objectives for ML embedding
- Assessing the current condition of manufacturing operations and identifying areas for improvement
- Developing a solid data management plan for enabling ML integration
By following these best practices, manufacturers can maximize the benefits of ML integration. Benefits you will see, as they will be great competitors in the manufacturing business.
Implementing best practices on ML integration is essential as the manufacturing industry continues to evolve. It’s vital for staying relevant and achieving sustainable success.
Best Practice | Description |
Data Preparation | Preparing ML data quality that integrates with the data environment |
Model Selection | Choosing the appropriate models for machine learning-based manufacturing operations |
Deployment Strategies | How to Deploy ML models to Fuel the Business |
Metrics Success Measurement
To understand whether machine learning is effective in manufacturing, we need performance metrics. These metrics allow us to track success. We search for KPIs that are in alignment with your objectives.
Some key performance indicators in manufacturing include production efficiency, product quality, and equipment uptime. They give us an overview of how machine learning is changing our work by tracking these. It helps us to decide the better ways to improve.
Key Performance Indicators
Choosing appropriate key performance indicators is critical. We need to consider what matters to us as an organization. Here are some examples:
- Production volume
- Product quality ratings
- Equipment uptime and downtime
- Supply chain efficiency
ROI Assessment Methods
So, to see if machine learning is a good idea we use different methods. Cost-benefit > payback period analysis, are common. These enable us to check if machine learning benefits exceed costs.
Strategies for Fractional Improvement
Once we establish performance metrics and key performance indicators (KPIs) we can begin to optimize. We monitor our metrics, identify areas for improvement, and adjust. This helps us to maintain a good performance.
These defining metrics of success will ensure our machine learning investments pay dividends. This keeps improving the quality of our work.
Key Performance Indicators | Target Values | Actual Values |
Production Volume | 1000 units/day | 900 units/day |
Product Quality Ratings | 95% | 92% |
Equipment Uptime | 95% | 90% |
Conclusion
Machine learning is revolutionizing the manufacturing industry. It introduces new efficiencies, quality, and sustainability. It aids in predictive maintenance, quality control, supply chain optimization, and energy management.
Advanced analytics, edge computing, and AI-ML systems will define the future of making things. And those technologies will deliver better results to manufacturers, save costs, and protect the planet.
To succeed, manufacturers must apply machine learning judiciously. They should prioritize seamless technical integration, training their people and ensuring data security. Machine learning in manufacturing: Towards a sustainable, innovative, and efficient future
FAQ
What is the role of machine learning in modern manufacturing?
Through forecasting, machine learning has revolutionized manufacturing. So it enables predictive maintenance and quality control. It also automates production lines and streamlines supply chains. This helps production, ensures, and focuses on product quality.
How to Improve software quality assurance and find defects with machine learning?
Quality checks can be improved through machine learning. It employs visual inspections and real-time monitoring. This can identify and classify defects for better product quality and waste reduction.
Why machine learning for predictive maintenance?
Machine learning-based predictive maintenance systems monitor the health of equipment. They predict when things will break down and when to do maintenance. This reduces downtime, improves the performance of the equipment, and lowers maintenance costs.
How Does Machine Learning Optimize Energy Management and Sustainability?
Machine learning analyzes patterns of energy use to identify potential energy savings. The use of AI helps in resource utilization and reduces environmental impacts. This renders manufacturing sustainable.
What are some of the main hurdles your technology faces in implementing machine learning within the manufacturing space?
There are bigger obstacles like technical integration, getting employees on board, and protecting sensitive data. With a robust plan and best practices, overcoming these is the key to success.
How can manufacturers know whether their machine-learning deployments have been successful?
To test whether machine learning is beneficial, check the KPIs and ROI. Learn and continuously improve: use a test and keep improving approach. Checking and tuning these metrics regularly is key to getting the best out of machine learning.